I design machine learning algorithms that try to solve some of today's most challenging problems in computer science and statistics.
I adapt ideas from physics and the statistical sciences, and use them in algorithms that can be applied to areas such as: bioinformatics, artificial intelligence, pattern recognition, document information retrieval, and human-computer interaction.
Click on the following topics to see research descriptions and some papers:-
Nonparametric Bayes | - | powerful nonparametric text/document modelling |
Variational Bayesian Methods | - | approximate Bayesian learning and inference |
Bioinformatics | - | microarray analysis using variational Bayes |
Embedded Hidden Markov Models | - | a novel tool for time series inference |
Probabilistic Sensor Fusion | - | combining modalities using Bayesian graphical models |
Collaborators | - | people I have worked with |
Bioinformatics
With a wealth of data becoming available from gene microarray experiments, there is plenty of room for application of machine learning algorithms. My current work involves applying VB versions of feedback state-space models (see chapter 5 of my thesis) to microarray data to elucidate gene-gene interactions and new and exciting pathways for cell processes such as apoptosis and proliferation.
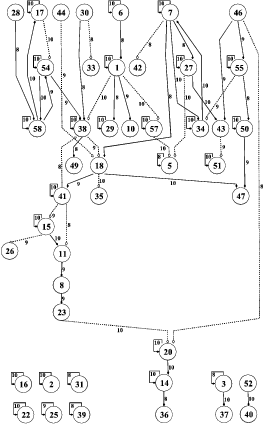
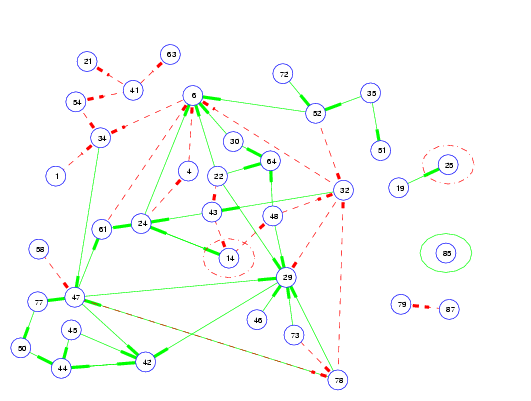
-
Beal, M.J., Falciani, F., Ghahramani Z., Rangel, C. and Wild, D.L.
A Bayesian Approach to Reconstructing Genetic Regulatory Networks with Hidden Factors
In Bioinformatics 21:349-356, 2005.
[abstract] advance access Sept 7th [pdf] supplementary data page Classical and Bayesian approaches to reconstructing genetic regulatory networks (28th July 2004)
A poster presented at the 12th International Conference on Intelligent Systems for Molecular Biology (ISMB'04) in Edinburgh on 02/08/04.
[pdf] [ps.gz]