Anomaly Detection from Big and Complex Data
Collaborators: Prof. Shambhu Upadhyaya, Prof. Hung Ngo, Dr. Oliver Kennedy, Dr. Dimitrios Koutsonikolas (UB CSE), Prof. Raghav Rao (UTSA), Dr. Rohit Valecha (UTSA)
Funding: NSF SaTC (Insider Threat Detection), NSF SaTC (Rumor Propagation)
Insider Threat Detection - Exploiting Data Relationships to Detect Insider Attacks
Insider attacks present an extremely serious, pervasive and costly security problem under critical domains such as national defense and financial and banking sector. Accurate insider threat detection has proved to be a very challenging problem. This project explores detecting insider threats in a banking environment by analyzing database searches.
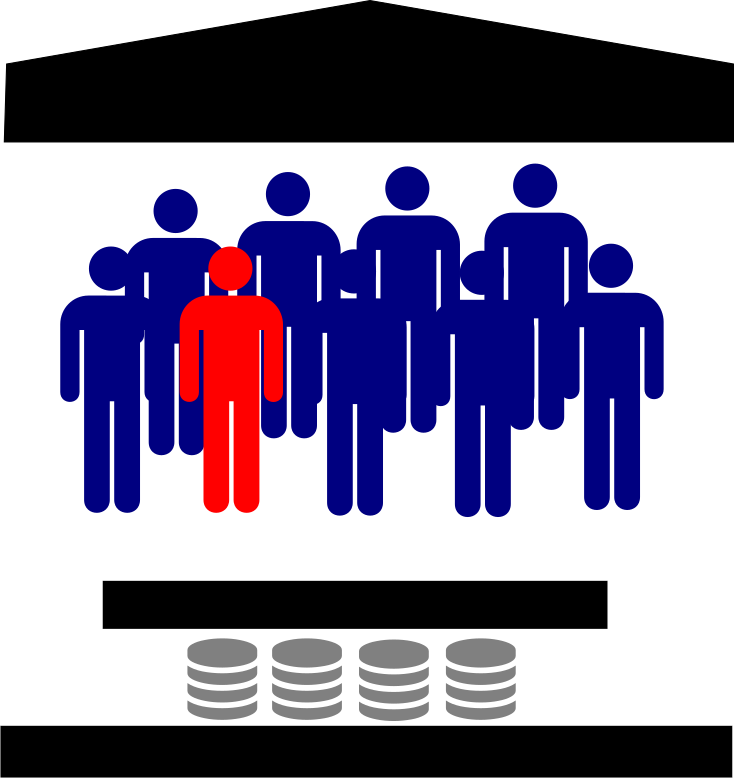
This research addresses the challenge by formulating and devising machine learning-based solutions to the insider attack problem on relational database management systems (RDBMS), which are ubiquitous and are highly susceptible to insider attacks. In particular, the research uses a new general model for database provenance, which captures both the data values accessed or modified by a user's activity and summarizes the computational path and the underlying relationship between those data values. The provenance model leads naturally to a way to model user activities by labeled hypergraph distributions and by a Markov network whose factors represent the data relationships. The key tradeoff being studied theoretically is between the expressivity and the complexity of the provenance model. The research results are validated and evaluated by intimately collaborating with a large financial institution to build a prototype insider threat detection engine operating on its existing operational RDBMS. In particular, with the help of the security team from the financial institution, the research team addresses database performance, learning scalability, and software tool development issues arising during the evaluation and deployment of the system.
Understanding Rumor Propagation in Social Networks
One prominent threat action (attack vector) in social cyber-attacks is the use of rumors. We are developing methods that integrate social science and computer science methods and new analysis techniques to process large-scale tweet data for detection and alerting of rumors. One of the key component of such research is the availability of a testbed for validating methods. In the context of social media, this requires a realistic network structure and models for simulating the spread of information, including rumors. We have developed statistical generative models for graphs that are able to capture the properties of graphs/networks that occur in real world [IEEE BigData, 2015].
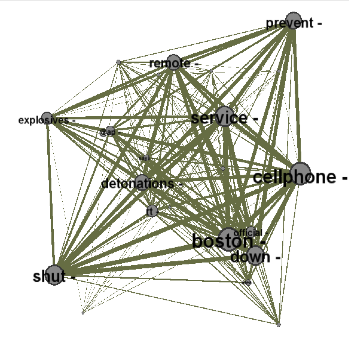
While in the past, focus was on generating graphs which follow general laws, such as the power law for degree distribution, current models have the ability to learn from observed graphs and generate synthetic approximations. The primary emphasis of existing models has been to closely match different properties of a single observed graph. Such models, though stochastic, tend to generate samples which do not have significant variance in terms of the various graph properties. We argue that in many cases real graphs are part of a population (e.g., networks sampled at various time points, social networks for individual schools, healthcare networks for different geographic regions, etc.). Graphs in a population exhibit variance. However, existing models are not designed to model this variance, which could lead to issues such as overfitting. We propose a graph generative model that focuses on matching the properties of real graphs and the natural variance expected for the corresponding population. The proposed model adopts a mixture-model strategy to expand the expressiveness of Kronecker product based graph models (KPGM), while building upon the two strengths of KPGM, viz., ability to model several key properties of graphs and to scale to massive graph sizes using its elegant fractal growth based formulation. The proposed model, called x-Kronecker Product Graph Model, or xKPGM, allows scalable learning from observed graphs and generates samples that match the mean and variance of several salient graph properties.